How Data-Driven Quality Checks Improve Product Reliability
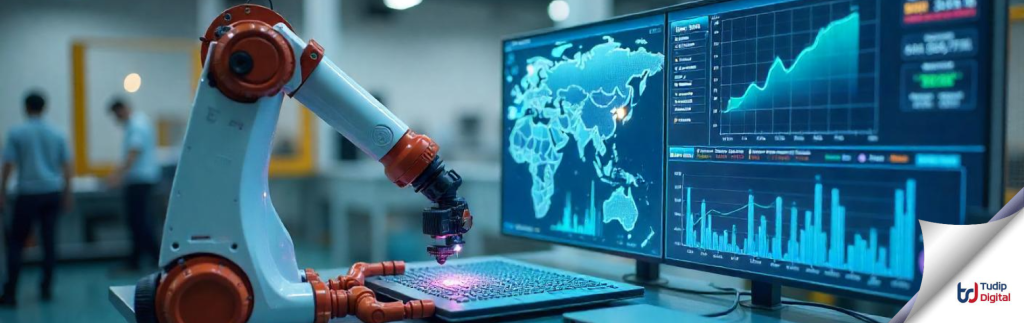
Introduction Manufacturers today operate in a highly competitive environment where product quality and reliability directly impact profitability and customer trust. Traditional quality control methods, which relied on manual inspections and reactive maintenance, often led to delays, inconsistencies, and high production costs. The shift toward data-driven quality checks has transformed manufacturing, enabling businesses to predict defects, optimize processes, and ensure consistency at scale. At Tudip Technologies, we understand the growing need for automated, data-driven quality assurance solutions. By integrating predictive analytics, real-time monitoring, and AI-powered inspections, we help manufacturers improve operational efficiency and minimize production flaws. This blog explores how data-driven quality checks enhance product reliability and process efficiency while addressing industry challenges. Why Traditional Quality Checks Are No Longer Sufficient For decades, manufacturers have relied on random sampling, manual inspections, and periodic audits to ensure quality. However, these methods come with significant drawbacks: Delayed Issue Detection: Problems are often identified too late, leading to costly rework. Human Error: Subjective assessments lead to inconsistencies. Limited Scalability: As production volumes increase, manual checks become inefficient. Higher Operational Costs: Frequent breakdowns and quality failures increase overall production expenses. By shifting to real-time, data-driven quality assurance, manufacturers can reduce defects, production downtime, and material waste, ensuring a more efficient and cost-effective production cycle. How Data-Driven Quality Checks Enhance Product Reliability 1. AI-Powered Defect Detection for Faster Decision-Making The integration of computer vision and AI-powered image analysis allows manufacturers to detect minute product defects with a higher level of accuracy. AI models trained on historical defect patterns can: Identify flaws in surface textures, dimensions, and material integrity faster than human inspectors. Improve precision by reducing false positives and missed defects. Continuously learn from past defect data to enhance detection accuracy over time. For manufacturers operating in automotive, electronics, and precision manufacturing, AI-powered inspections significantly improve product quality consistency and customer satisfaction. 2. Real-Time Quality Monitoring & Predictive Maintenance Instead of reacting to production failures, manufacturers can monitor quality metrics in real time through IoT sensors and predictive analytics. This allows businesses to: Detect anomalies in production lines before they cause defects. Optimize machine performance to reduce unexpected breakdowns. Reduce unplanned downtime by scheduling proactive maintenance based on real-time data insights. By integrating machine learning-driven monitoring systems, manufacturers can increase equipment lifespan, lower maintenance costs, and maintain production efficiency. 3. Automated Process Optimization to Reduce Variability Variations in production workflows often lead to inconsistent quality levels. Through automated data analysis, manufacturers can: Identify variability in production batches and standardize processes accordingly. Optimize operational parameters in real time for greater uniformity. Reduce scrap rates by identifying root causes of inconsistencies. Tudip Technologies has worked with global manufacturing clients to implement real-time data-driven process optimizations, resulting in higher product reliability and lower defect rates. 4. Historical Data Analysis for Continuous Improvement Manufacturers often need to analyze long-term trends to improve quality assurance. Cloud-based data storage and AI-driven analytics help: Identify recurring quality issues across different production runs. Compare past and present defect trends to refine quality control processes. Automate compliance reporting, ensuring adherence to industry standards. By leveraging big data analytics, businesses can develop data-backed strategies for long-term improvements in quality, compliance, and operational efficiency. Industry Impact: Real-World Applications of Data-Driven Quality Checks Electronics Manufacturing High-precision electronics require defect-free components to function reliably. Data-driven quality assurance ensures: Automated PCB inspections with AI-based optical analysis. Material integrity testing to detect potential failures. Automated troubleshooting to prevent device malfunctions. Automotive Industry Car manufacturers benefit from real-time defect tracking and predictive maintenance, reducing recalls and increasing vehicle safety. Chassis and frame inspections for structural integrity. AI-powered failure prediction in critical components like brakes and engines. Supplier quality checks to ensure consistency in parts procurement. Pharmaceutical Manufacturing In the pharmaceutical industry, precision and compliance are critical. Data-driven quality checks help: Detects anomalies in drug formulations to ensure patient safety. Monitor environmental factors affecting production (temperature, humidity). Automate batch testing to maintain regulatory compliance. Challenges in Implementing Data-Driven Quality Control While data-driven quality checks provide significant benefits, manufacturers may face challenges in: Data Integration: Combining quality data from multiple sources can be complex. Initial Investment Costs: Implementing AI and IoT-driven monitoring requires infrastructure upgrades. Skill Gaps: Managing data analytics and AI models requires trained professionals. However, Tudip Technologies helps businesses navigate these challenges by offering scalable, cost-effective, and customized solutions to integrate real-time analytics and AI-driven quality control systems. Looking Ahead: The Future of Quality Assurance in Manufacturing The future of quality control will be shaped by: Autonomous defect detection systems that eliminate manual inspections. AI-powered root cause analysis, reducing long-term quality issues. Edge computing in quality monitoring, enabling real-time decision-making at production sites. As the industry moves toward smart factories and Industry 4.0, manufacturers must adopt data-driven quality control strategies to stay competitive. Conclusion The shift to data-driven quality checks is transforming manufacturing by improving defect detection, predictive maintenance, automated process optimization, and long-term product reliability. Companies leveraging AI, IoT, and real-time analytics are setting new benchmarks for efficiency, consistency, and cost-effectiveness. At Tudip Technologies, we specialize in integrating data analytics, AI-powered inspections, and real-time monitoring to enhance manufacturing quality control. If you’re looking to improve product reliability and reduce defects, contact us at info@tudip.com to explore tailored solutions for your industry.
Optimizing Manufacturing Resource Allocation Through Data-Driven Decision Support
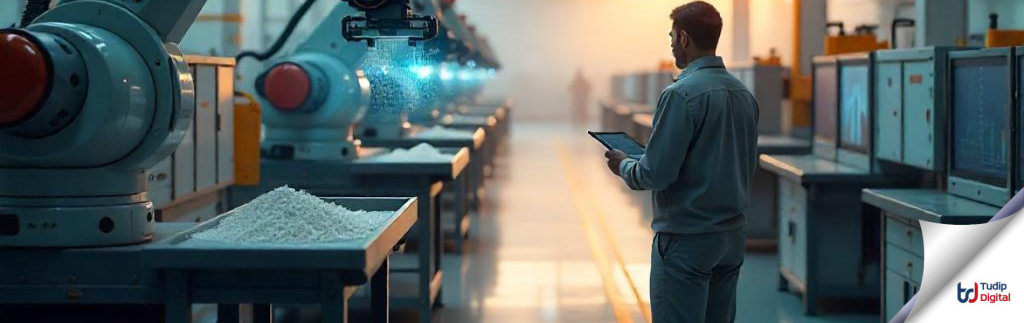
Introduction Manufacturing success depends on the efficient allocation of resources—materials, labor, machinery, and energy. Traditionally, manufacturers relied on historical data and manual processes to plan their resource usage. However, with increasing supply chain complexities, fluctuating market demands, and the need for greater efficiency, these traditional approaches are no longer sufficient. Data-driven decision support enables manufacturers to optimize resource allocation by leveraging real-time analytics, predictive modeling, and AI-driven insights. With better visibility and informed decision-making, companies can reduce costs, improve operational efficiency, and enhance scalability. This blog explores how data-driven strategies can transform resource allocation in manufacturing, ensuring businesses remain competitive and agile. In recent years, technology-driven firms have played a crucial role in modernizing manufacturing processes. With expertise in predictive analytics, AI, and cloud-based solutions, companies like Tudip Technologies have helped manufacturers transition from traditional planning to intelligent, automated decision-making frameworks that enhance agility and sustainability. Challenges in Traditional Resource Allocation 1. Limited Real-Time Visibility Most manufacturers lack instant access to operational data, making it difficult to track resource usage and efficiency. This often leads to overuse of raw materials, inefficient labor distribution, and unexpected machine downtimes. 2. Demand Forecasting Inaccuracy Traditional forecasting relies on past trends, making it challenging to predict sudden market shifts. This results in either overproduction, which leads to excessive inventory costs, or underproduction, which causes supply chain bottlenecks. 3. High Operational Costs Due to Inefficiencies Misallocation of resources leads to increased energy consumption, unnecessary labor costs, and excess waste, all of which negatively impact profitability. 4. Limited Scalability and Adaptability Manufacturers using static planning models struggle to adjust their production schedules dynamically, making it difficult to respond to sudden changes in raw material availability, labor shortages, or equipment failures. How Data-Driven Decision Support Transforms Resource Allocation 1. Real-Time Data Analytics for Smarter Decision-Making Cloud-based systems and IoT sensors collect real-time data on machine performance, labor productivity, and material flow. Centralized dashboards allow decision-makers to track key metrics and adjust resource allocation accordingly. 2. Predictive Analytics for Demand Planning AI-driven models analyze market trends, historical sales, and external factors to provide accurate demand forecasts. This ensures production aligns with actual consumer demand, reducing excess inventory and optimizing material procurement. 3. AI-Powered Production Scheduling AI models optimize production sequences by allocating resources based on real-time capacity and workload distribution. Machine learning algorithms predict equipment failures and schedule preventive maintenance, minimizing downtime. 4. Automation for Efficient Resource Allocation Automated workflows improve labor deployment, ensuring workers are assigned to high-priority tasks efficiently. AI-powered quality control systems identify defects early, reducing material wastage and improving product reliability. With deep expertise in data analytics, cloud technology, and AI-driven automation, Tudip Technologies has successfully collaborated with manufacturing firms to implement real-time tracking and predictive modeling solutions, helping them achieve greater operational accuracy and resource efficiency. Key Benefits of Data-Driven Resource Allocation 1. Improved Productivity and Efficiency Optimized resource planning eliminates unnecessary delays and supply chain bottlenecks. Automated workflows and real-time tracking enhance workforce efficiency. 2. Reduced Costs and Minimized Waste AI-driven insights prevent overuse of raw materials and reduce energy consumption. Better forecasting helps avoid excess inventory storage costs. 3. Greater Scalability and Flexibility Manufacturers can dynamically adjust production schedules based on market demand. Cloud-based platforms support seamless expansion without excessive infrastructure investments. 4. Enhanced Compliance and Sustainability Automated reporting ensures compliance with industry regulations on material usage and environmental impact. Data-driven sustainability initiatives help reduce carbon footprints and energy waste. Implementation Roadmap for Manufacturers Step 1: Assess Current Resource Utilization Conduct an operational audit to identify inefficiencies in material usage, workforce deployment, and production capacity. Analyze existing data sources to understand key performance gaps. Step 2: Integrate AI and Predictive Analytics Deploy machine learning models to optimize demand forecasting and production scheduling. Use predictive analytics for preventive maintenance and equipment health monitoring. Step 3: Automate Resource Allocation Processes Implement cloud-based ERP solutions for real-time tracking and automated decision-making. Introduce AI-powered scheduling systems to allocate labor and machinery more efficiently. Step 4: Monitor and Optimize Performance Set up dashboards for continuous performance tracking and resource utilization analysis. Use feedback loops to refine AI models and improve forecasting accuracy. Through collaborations with technology partners like Tudip Technologies, manufacturers have successfully implemented AI-based predictive analytics and automated workflows that enable real-time decision-making—reducing costs and improving supply chain efficiency. The Future of Data-Driven Resource Allocation 1. Digital Twin Technology for Real-Time Simulations Manufacturers can create virtual models of their production facilities to test different resource allocation strategies before implementing them in real operations. 2. AI-Powered Chatbots for Decision Support AI assistants can provide real-time recommendations on adjusting resource allocation based on production demands. 3. Cloud-Based Collaborative Resource Management Global manufacturing teams can collaborate in real time to adjust resource allocation across multiple facilities, optimizing supply chain logistics. Conclusion As manufacturing becomes more complex, data-driven decision support is no longer optional—it’s essential for maintaining efficiency, reducing costs, and staying competitive. By leveraging real-time analytics, predictive modeling, and AI-driven automation, manufacturers can optimize resource allocation and adapt to changing market conditions. Companies that embrace data-driven strategies will gain a competitive edge in productivity, sustainability, and operational agility. With experience in data analytics, cloud computing, and predictive intelligence, Tudip Technologies has played a crucial role in helping businesses streamline their resource allocation strategies, improve operational decision-making, and enhance manufacturing efficiency. For more insights on implementing data-driven solutions in manufacturing, contact info@tudip.com.
Reducing Downtime Through Predictive Maintenance

Unplanned downtime is one of the most significant challenges manufacturers face, costing millions annually in lost productivity and delayed orders.
Streamlining Manufacturing with Data-Driven Decisions
Introduction Manufacturing operations today are more complex than ever before. With the rapid pace of technological advancements and evolving market demands, manufacturers must find smarter ways to manage their production lines, inventory, and equipment. Traditional methods of oversight and decision-making can no longer keep up with these challenges. This is where data-driven decision-making steps in—a transformative approach that leverages real-time data and analytics to optimize manufacturing processes. By embracing data as a strategic tool, manufacturers can enhance efficiency, reduce waste, and maintain a competitive edge in the modern landscape. Key Benefits of Data-Driven Decisions in Manufacturing Improved Operational Efficiency Data-driven insights streamline processes by pinpointing inefficiencies and optimizing workflows. Real-time data enables quick adjustments to production lines, ensuring that operations remain agile and responsive to changing demands. Proactive Maintenance Instead of reacting to equipment breakdowns, manufacturers can use predictive analytics to foresee issues before they occur. This approach minimizes unplanned downtime, reduces maintenance costs, and extends the life of machinery. Enhanced Quality Control Data analytics allows manufacturers to monitor production quality in real time, identifying anomalies early in the process. This minimizes defects, reduces waste, and ensures that products consistently meet high standards. Optimized Inventory Management Accurate inventory tracking and demand forecasting are critical for maintaining balance. By analyzing data, manufacturers can ensure they have the right materials at the right time, reducing excess stock and avoiding delays caused by shortages. Applications of Data-Driven Decisions Predictive Maintenance to Reduce Downtime Unplanned downtime is one of the most costly challenges in manufacturing. Predictive maintenance uses sensors and data analytics to monitor equipment health, enabling timely interventions that prevent breakdowns. This approach reduces downtime by up to 30% and enhances overall productivity. Inventory Optimization for Cost Savings Data-driven tools help manufacturers align inventory levels with production needs. Analyzing trends and patterns ensures that materials are neither overstocked nor understocked, improving cost efficiency and keeping production running smoothly. Real-Time Quality Monitoring With data-driven insights, manufacturers can implement systems that detect defects as they happen. This immediate feedback loop allows for quick corrections, ensuring that products meet quality standards without the need for costly rework or waste. Workforce Productivity Analyzing production data can guide better workforce allocation, ensuring that the right number of workers are assigned to tasks at the right time. This reduces labor costs while maintaining peak productivity. Steps to Implement Data-Driven Decision-Making Establish Reliable Data Collection Systems Sensors and IoT devices are essential for collecting accurate, real-time data from machinery, production lines, and inventory systems. These technologies form the foundation for effective decision-making. Adopt Analytics Tools for Actionable Insights Invest in advanced analytics platforms that transform raw data into meaningful insights. These tools help manufacturers identify trends, predict outcomes, and make informed decisions that drive efficiency. Train Teams to Leverage Data Technology is only as effective as the people using it. Provide training to your teams on interpreting data and applying insights to their daily operations, ensuring they are equipped to make data-driven decisions confidently. Create Feedback Loops for Continuous Improvement Regularly review performance metrics and refine processes based on data insights. This iterative approach allows manufacturers to stay agile and achieve sustained growth over time. Conclusion The complexities of modern manufacturing demand smarter, faster, and more efficient decision-making. Data-driven decisions offer a powerful way to address these challenges, providing actionable insights that improve operational efficiency, enhance quality, and reduce costs. By adopting a strategic approach to data, manufacturers can not only overcome current hurdles but also position themselves for long-term success. The future of manufacturing is data-driven—start your journey toward streamlined operations today. Why Tudip? Tudip Technologies specializes in delivering tailored, data-driven solutions that empower manufacturers to optimize their operations. With expertise in predictive maintenance, real-time analytics, and inventory management systems, we help businesses reduce downtime, enhance quality, and achieve measurable results. Our advanced analytics platforms and IoT integration capabilities ensure seamless implementation and continuous improvement, making Tudip a trusted partner for manufacturers seeking operational excellence.